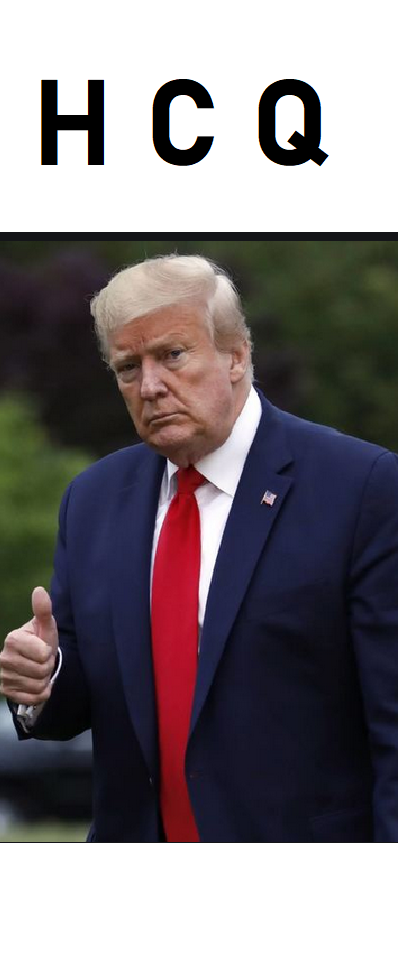
| 10.1148/radiol.2020201491
http://scihub22266oqcxt.onion/10.1148/radiol.2020201491
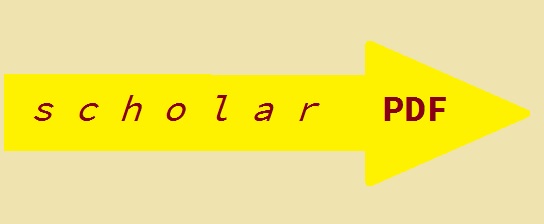 32339081!7233483!32339081
free
free
free
Warning: file_get_contents(https://eutils.ncbi.nlm.nih.gov/entrez/eutils/elink.fcgi?dbfrom=pubmed&id=32339081&cmd=llinks): Failed to open stream: HTTP request failed! HTTP/1.1 429 Too Many Requests
in C:\Inetpub\vhosts\kidney.de\httpdocs\pget.php on line 215
|  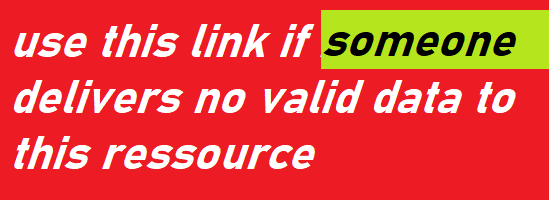
Deprecated: Implicit conversion from float 235.6 to int loses precision in C:\Inetpub\vhosts\kidney.de\httpdocs\pget.php on line 534
Deprecated: Implicit conversion from float 235.6 to int loses precision in C:\Inetpub\vhosts\kidney.de\httpdocs\pget.php on line 534
Deprecated: Implicit conversion from float 235.6 to int loses precision in C:\Inetpub\vhosts\kidney.de\httpdocs\pget.php on line 534
Deprecated: Implicit conversion from float 235.6 to int loses precision in C:\Inetpub\vhosts\kidney.de\httpdocs\pget.php on line 534
Deprecated: Implicit conversion from float 235.6 to int loses precision in C:\Inetpub\vhosts\kidney.de\httpdocs\pget.php on line 534
Deprecated: Implicit conversion from float 269.2 to int loses precision in C:\Inetpub\vhosts\kidney.de\httpdocs\pget.php on line 534
Deprecated: Implicit conversion from float 269.2 to int loses precision in C:\Inetpub\vhosts\kidney.de\httpdocs\pget.php on line 534
Deprecated: Implicit conversion from float 269.2 to int loses precision in C:\Inetpub\vhosts\kidney.de\httpdocs\pget.php on line 534
Deprecated: Implicit conversion from float 269.2 to int loses precision in C:\Inetpub\vhosts\kidney.de\httpdocs\pget.php on line 534
Deprecated: Implicit conversion from float 269.2 to int loses precision in C:\Inetpub\vhosts\kidney.de\httpdocs\pget.php on line 534
Deprecated: Implicit conversion from float 269.2 to int loses precision in C:\Inetpub\vhosts\kidney.de\httpdocs\pget.php on line 534
Deprecated: Implicit conversion from float 269.2 to int loses precision in C:\Inetpub\vhosts\kidney.de\httpdocs\pget.php on line 534
Warning: imagejpeg(C:\Inetpub\vhosts\kidney.de\httpdocs\phplern\32339081.jpg): Failed to open stream: No such file or directory in C:\Inetpub\vhosts\kidney.de\httpdocs\pget.php on line 117
Radiology 2020 ; 296 (3): E156-E165 Nephropedia Template TP
gab.com Text
Twit Text FOAVip
Twit Text #
English Wikipedia
|
Artificial Intelligence Augmentation of Radiologist Performance in Distinguishing COVID-19 from Pneumonia of Other Origin at Chest CT #MMPMID32339081Bai HX; Wang R; Xiong Z; Hsieh B; Chang K; Halsey K; Tran TML; Choi JW; Wang DC; Shi LB; Mei J; Jiang XL; Pan I; Zeng QH; Hu PF; Li YH; Fu FX; Huang RY; Sebro R; Yu QZ; Atalay MK; Liao WHRadiology 2020[Sep]; 296 (3): E156-E165 PMID32339081show ga
Background Coronavirus disease 2019 (COVID-19) and pneumonia of other diseases share similar CT characteristics, which contributes to the challenges in differentiating them with high accuracy. Purpose To establish and evaluate an artificial intelligence (AI) system for differentiating COVID-19 and other pneumonia at chest CT and assessing radiologist performance without and with AI assistance. Materials and Methods A total of 521 patients with positive reverse transcription polymerase chain reaction results for COVID-19 and abnormal chest CT findings were retrospectively identified from 10 hospitals from January 2020 to April 2020. A total of 665 patients with non-COVID-19 pneumonia and definite evidence of pneumonia at chest CT were retrospectively selected from three hospitals between 2017 and 2019. To classify COVID-19 versus other pneumonia for each patient, abnormal CT slices were input into the EfficientNet B4 deep neural network architecture after lung segmentation, followed by a two-layer fully connected neural network to pool slices together. The final cohort of 1186 patients (132 583 CT slices) was divided into training, validation, and test sets in a 7:2:1 and equal ratio. Independent testing was performed by evaluating model performance in separate hospitals. Studies were blindly reviewed by six radiologists without and then with AI assistance. Results The final model achieved a test accuracy of 96% (95% confidence interval [CI]: 90%, 98%), a sensitivity of 95% (95% CI: 83%, 100%), and a specificity of 96% (95% CI: 88%, 99%) with area under the receiver operating characteristic curve of 0.95 and area under the precision-recall curve of 0.90. On independent testing, this model achieved an accuracy of 87% (95% CI: 82%, 90%), a sensitivity of 89% (95% CI: 81%, 94%), and a specificity of 86% (95% CI: 80%, 90%) with area under the receiver operating characteristic curve of 0.90 and area under the precision-recall curve of 0.87. Assisted by the probabilities of the model, the radiologists achieved a higher average test accuracy (90% vs 85%, Delta = 5, P < .001), sensitivity (88% vs 79%, Delta = 9, P < .001), and specificity (91% vs 88%, Delta = 3, P = .001). Conclusion Artificial intelligence assistance improved radiologists' performance in distinguishing coronavirus disease 2019 pneumonia from non-coronavirus disease 2019 pneumonia at chest CT. (c) RSNA, 2020 Online supplemental material is available for this article.|*Artificial Intelligence[MESH]|*Radiologists/standards/statistics & numerical data[MESH]|Adolescent[MESH]|Adult[MESH]|Aged[MESH]|Aged, 80 and over[MESH]|Betacoronavirus[MESH]|COVID-19[MESH]|Child[MESH]|Child, Preschool[MESH]|China[MESH]|Coronavirus Infections/*diagnostic imaging[MESH]|Diagnosis, Differential[MESH]|Female[MESH]|Humans[MESH]|Infant[MESH]|Infant, Newborn[MESH]|Lung/diagnostic imaging[MESH]|Male[MESH]|Middle Aged[MESH]|Pandemics[MESH]|Philadelphia[MESH]|Pneumonia, Viral/*diagnostic imaging[MESH]|Pneumonia/diagnostic imaging[MESH]|Radiography, Thoracic[MESH]|Retrospective Studies[MESH]|Rhode Island[MESH]|SARS-CoV-2[MESH]|Sensitivity and Specificity[MESH]|Tomography, X-Ray Computed/*methods[MESH]
  
DeepDyve Pubget Overpricing | 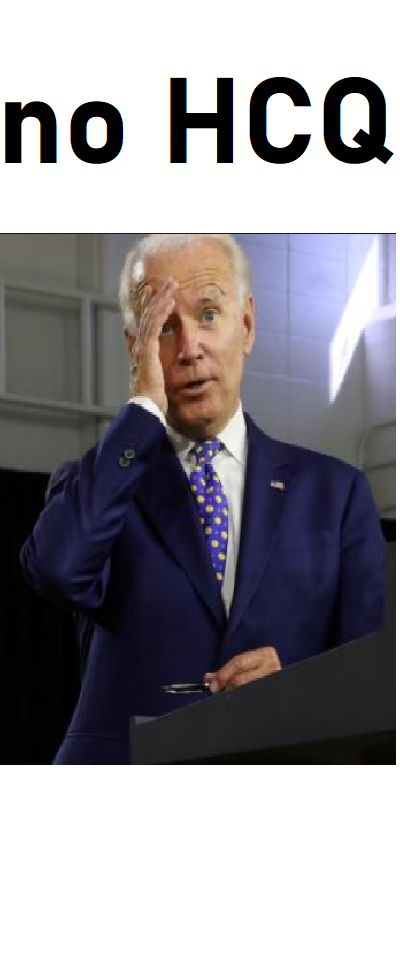
|