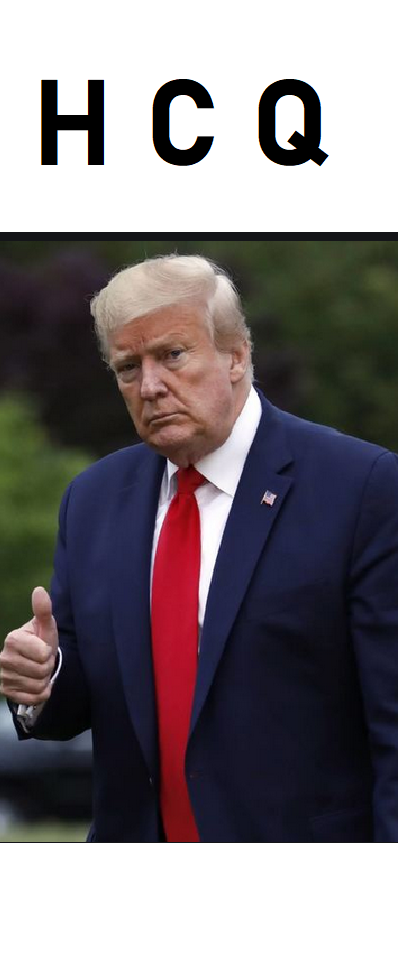
| 10.1371/journal.pone.0140123
http://scihub22266oqcxt.onion/10.1371/journal.pone.0140123
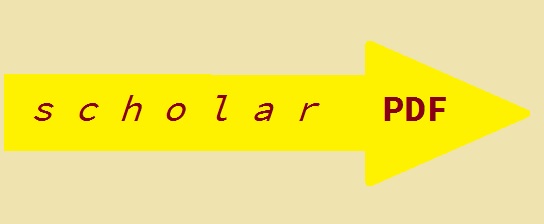 C4604143!4604143!26461492
free
free
free
Warning: file_get_contents(https://eutils.ncbi.nlm.nih.gov/entrez/eutils/elink.fcgi?dbfrom=pubmed&id=26461492&cmd=llinks): Failed to open stream: HTTP request failed! HTTP/1.1 429 Too Many Requests
in C:\Inetpub\vhosts\kidney.de\httpdocs\pget.php on line 215
|  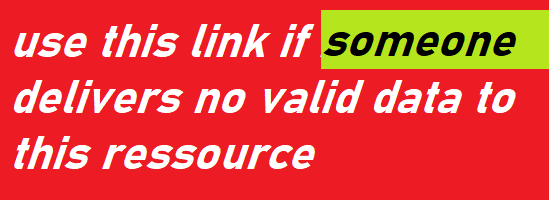
Deprecated: Implicit conversion from float 217.6 to int loses precision in C:\Inetpub\vhosts\kidney.de\httpdocs\pget.php on line 534
Deprecated: Implicit conversion from float 217.6 to int loses precision in C:\Inetpub\vhosts\kidney.de\httpdocs\pget.php on line 534
Deprecated: Implicit conversion from float 217.6 to int loses precision in C:\Inetpub\vhosts\kidney.de\httpdocs\pget.php on line 534
Deprecated: Implicit conversion from float 217.6 to int loses precision in C:\Inetpub\vhosts\kidney.de\httpdocs\pget.php on line 534
Deprecated: Implicit conversion from float 217.6 to int loses precision in C:\Inetpub\vhosts\kidney.de\httpdocs\pget.php on line 534
Deprecated: Implicit conversion from float 217.6 to int loses precision in C:\Inetpub\vhosts\kidney.de\httpdocs\pget.php on line 534
Deprecated: Implicit conversion from float 217.6 to int loses precision in C:\Inetpub\vhosts\kidney.de\httpdocs\pget.php on line 534
Deprecated: Implicit conversion from float 251.2 to int loses precision in C:\Inetpub\vhosts\kidney.de\httpdocs\pget.php on line 534
Warning: imagejpeg(C:\Inetpub\vhosts\kidney.de\httpdocs\phplern\26461492.jpg): Failed to open stream: No such file or directory in C:\Inetpub\vhosts\kidney.de\httpdocs\pget.php on line 117
PLoS+One 2015 ; 10 (10): � Nephropedia Template TP
gab.com Text
Twit Text FOAVip
Twit Text #
English Wikipedia
|
Superiority of Classification Tree versus Cluster, Fuzzy and Discriminant Models in a Heartbeat Classification System #MMPMID26461492Krasteva V; Jekova I; Leber R; Schmid R; Ab�cherli RPLoS One 2015[]; 10 (10): � PMID26461492show ga
This study presents a 2-stage heartbeat classifier of supraventricular (SVB) and ventricular (VB) beats. Stage 1 makes computationally-efficient classification of SVB-beats, using simple correlation threshold criterion for finding close match with a predominant normal (reference) beat template. The non-matched beats are next subjected to measurement of 20 basic features, tracking the beat and reference template morphology and RR-variability for subsequent refined classification in SVB or VB-class by Stage 2. Four linear classifiers are compared: cluster, fuzzy, linear discriminant analysis (LDA) and classification tree (CT), all subjected to iterative training for selection of the optimal feature space among extended 210-sized set, embodying interactive second-order effects between 20 independent features. The optimization process minimizes at equal weight the false positives in SVB-class and false negatives in VB-class. The training with European ST-T, AHA, MIT-BIH Supraventricular Arrhythmia databases found the best performance settings of all classification models: Cluster (30 features), Fuzzy (72 features), LDA (142 coefficients), CT (221 decision nodes) with top-3 best scored features: normalized current RR-interval, higher/lower frequency content ratio, beat-to-template correlation. Unbiased test-validation with MIT-BIH Arrhythmia database rates the classifiers in descending order of their specificity for SVB-class: CT (99.9%), LDA (99.6%), Cluster (99.5%), Fuzzy (99.4%); sensitivity for ventricular ectopic beats as part from VB-class (commonly reported in published beat-classification studies): CT (96.7%), Fuzzy (94.4%), LDA (94.2%), Cluster (92.4%); positive predictivity: CT (99.2%), Cluster (93.6%), LDA (93.0%), Fuzzy (92.4%). CT has superior accuracy by 0.3?6.8% points, with the advantage for easy model complexity configuration by pruning the tree consisted of easy interpretable ?if-then? rules.�
  
DeepDyve Pubget Overpricing | 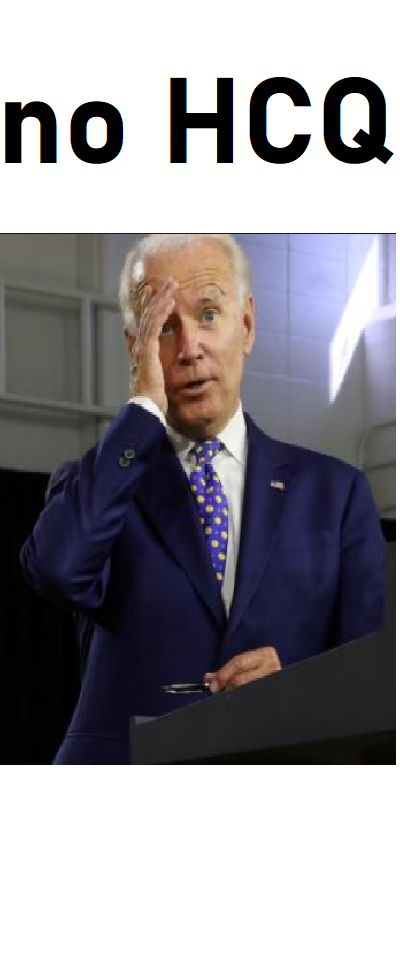
|